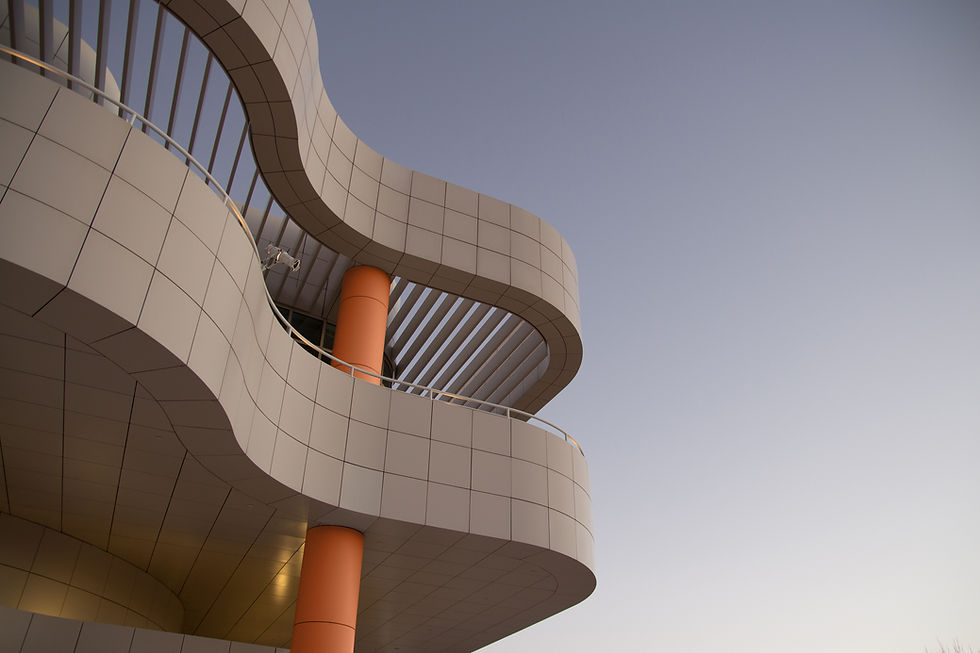
The Role of Natural Language Processing (NLP) in Pharmacovigilance
Jul 24, 2024
3 min read
2
37
0
Pharmacovigilance, the science of detecting, assessing, and preventing adverse effects of pharmaceutical products, has become a crucial aspect of the healthcare industry. With the increasing volume of data generated from various sources, including electronic health records, social media, and clinical trials, the need for efficient and accurate analysis tools has become more pressing than ever. This is where Natural Language Processing (NLP) comes into play. In this blog, we will explore the role of NLP in pharmacovigilance and how it is revolutionizing the field.
The Challenge of Pharmacovigilance:
Pharmacovigilance involves the monitoring of adverse drug reactions (ADRs) and other safety issues associated with pharmaceutical products. The process is complex, time-consuming, and requires the analysis of large amounts of data from various sources. Traditional methods of pharmacovigilance rely on manual review of reports, which can be prone to errors, biased, and slow. The sheer volume of data generated from various sources makes it difficult for humans to analyze and identify patterns, leading to delayed detection of safety issues.
The Power of NLP in Pharmacovigilance:
NLP, a subfield of artificial intelligence, is designed to enable computers to understand, interpret, and generate human language. In the context of pharmacovigilance, NLP can be used to analyze large volumes of unstructured data, such as text reports, social media posts, and clinical trial data, to identify patterns and trends that may indicate safety issues.
NLP can be applied in various ways in pharmacovigilance, including:
Text Mining: NLP can be used to extract relevant information from unstructured text data, such as adverse event reports, to identify patterns and trends that may indicate safety issues.
Sentiment Analysis: NLP can be used to analyze social media posts and online reviews to identify sentiment patterns that may indicate safety concerns.
Information Extraction: NLP can be used to extract relevant information from clinical trial data, such as patient demographics, medication history, and adverse event reports.
Machine Learning: NLP can be used to train machine learning models to identify patterns and trends in data that may indicate safety issues.
Benefits of NLP in Pharmacovigilance:
Improved Accuracy: NLP can analyze large volumes of data quickly and accurately, reducing the risk of human error.
Increased Efficiency: NLP can automate the analysis of data, freeing up human resources to focus on higher-level tasks.
Enhanced Insights: NLP can identify patterns and trends in data that may not be apparent to humans, providing enhanced insights into safety issues.
Faster Detection: NLP can detect safety issues faster than traditional methods, enabling quicker response times and reducing the risk of harm to patients.
Real-World Applications:
Several organizations are already leveraging NLP in pharmacovigilance, including:
The Food and Drug Administration (FDA): The FDA is using NLP to analyze large volumes of data from various sources, including social media and electronic health records, to identify safety issues.
Pharmaceutical Companies: Pharmaceutical companies, such as Pfizer and GlaxoSmithKline, are using NLP to analyze clinical trial data and identify patterns and trends that may indicate safety issues.
Healthcare Organizations: Healthcare organizations, such as hospitals and clinics, are using NLP to analyze electronic health records and identify safety issues.
Conclusion:
The role of NLP in pharmacovigilance is revolutionizing the field by enabling the efficient and accurate analysis of large volumes of data. By leveraging NLP, pharmacovigilance professionals can identify safety issues faster, improve accuracy, and enhance insights. As the volume of data continues to grow, the importance of NLP in pharmacovigilance will only continue to increase.